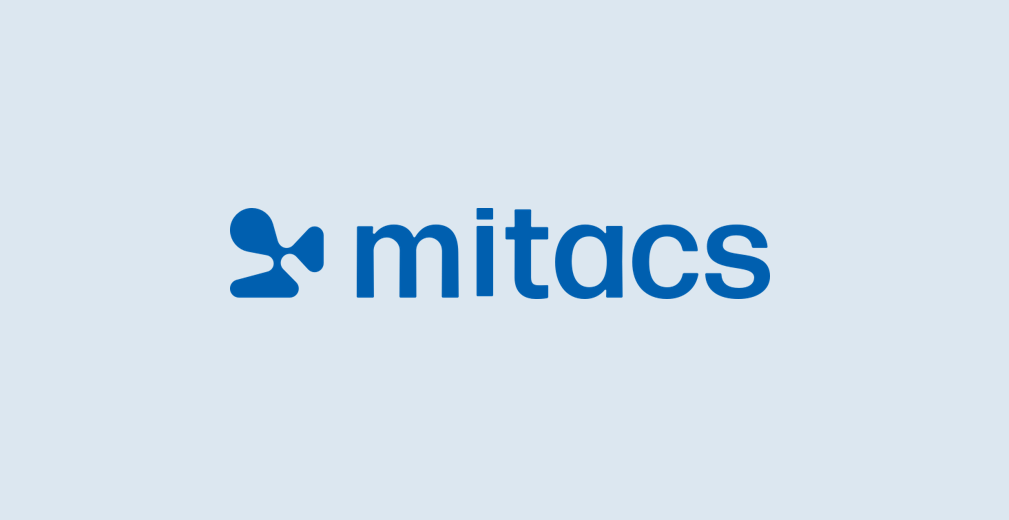
The project aims to use state-of-the-art machine learning techniques to perform model validation. In particular, the intern will validate outcomes from risk assessment models for loan portfolios. The results will be employed to further the efficiency of ATB’s internal stress testing models. The benefit for ATB financial will be the possibility to detect subsamples for […]
Read More